Here is the English translation of the text, with the specified terms translated as instructed:
The end of the search, the beginning of the research.
Author: One Useful Thing
Compiled by: TechFlow
Over the past weekend, we caught a glimpse of the future. For a long time, I have been discussing two important revolutions in AI: the rise of Autonomous Agents and the development of powerful Reasoners since OpenAI introduced the o1 model. These two technological paths have now converged, giving rise to an astonishing result - AI systems can not only conduct research with the depth and detail of human experts, but also complete it at machine speed. This convergence is represented by OpenAI's Deep Research, which paints a picture of the future. However, to understand why all this is important, we need to start from the basics: Reasoners and Agents.
Reasoners
In the past few years, when you used a chatbot, its work was usually very simple: you input a question, and the system would generate a response word-by-word (or more accurately, Token-by-Token). Since AI can only "think" while generating these Tokens, researchers have developed many techniques to enhance their reasoning abilities. For example, by prompting the AI to "reason step-by-step before answering", known as Chain-of-Thought Prompting, significantly improves the AI's performance.
The emergence of Reasoners automates this process. Before answering a question, the system will first generate "Thought Tokens" (i.e., reasoning steps), and then provide the final answer. This approach brings two important breakthroughs.
First, AI companies can train Reasoners by example from excellent problem solvers, making the AI's "thinking" process more efficient. This training method can generate higher-quality reasoning chains than human prompts, allowing Reasoners to solve more complex problems, especially in areas where traditional chatbots have performed poorly, such as mathematics and logic.
Secondly, a key feature of Reasoners is that the longer they "think", the higher the quality of the answer (although the rate of improvement will gradually slow down over time). This is particularly important because the only way to improve AI performance in the past was to train larger-scale models, which requires a lot of data and resources. Reasoners show that by simply generating more reasoning steps when answering a question (i.e., computing during reasoning), the performance can be significantly improved without increasing model training resources.
Agents
Experts have not yet reached a consensus on the definition of AI Agents. However, we can simply understand them as "AI systems that are given goals and can autonomously complete them". Currently, major AI labs are fiercely competing to develop general-purpose Agents - systems that can handle any task. I have mentioned some early examples, such as Devin and Claude, which have certain computer operation capabilities. Recently, OpenAI has just released Operator, which may be the most sophisticated general-purpose Agent to date.
The video (processed at 16x speed) demonstrates the great potential and current limitations of general-purpose Agents. I gave Operator the task of reading my latest Substack post on the OneUsefulThing platform, then accessing Google ImageFX to design a suitable image, and finally downloading it to send to me. Initially, Operator performed very well - it accurately found my website, read the article, navigated to ImageFX (pausing for me to input login information), and successfully created an image. However, problems soon arose, mainly in two aspects: one is that Operator was prevented from downloading the file by OpenAI's security restrictions, and the other is that chaos occurred during the task execution. The Agent tried various solutions, such as copying to the clipboard, generating direct links, and even delving into the website's source code. However, none of these attempts succeeded - some due to OpenAI's browser limitations, and others due to the Agent's misunderstanding of the task. Observing this persistent yet ultimately failed attempt reveals the current system's limitations and raises questions about how Agents will cope with obstacles in the real world.
Deep Research
OpenAI's Deep Research is a domain-specific Agent focused on the research field. It is based on OpenAI's yet-to-be-released o3 Reasoner system and is equipped with specialized tools and functions. This is one of the most impressive AI applications I've seen recently.
To demonstrate its capabilities, I set it a topic: when should a startup stop exploring and start scaling? This is a technically complex and controversial question in my research area. I asked Deep Research to investigate the relevant academic research, focusing on high-quality papers and randomized controlled trials (RCTs), and to address any definitional disputes and conflicts between common sense and research conclusions. Finally, it needs to present a comprehensive result for a graduate-level discussion.
At the start of the task, the AI raised several very insightful questions, and I further clarified my requirements. Then, OpenAI's o3 Reasoner system went to work. Throughout the process, you can clearly see its progress and "thought" process. You will find that the behavior of this AI system is very close to that of a researcher: it actively explores and delves into content that "interests it", and tries to solve problems (such as finding ways to bypass paywalls). The entire process took about five minutes.
In the end, I received a thirteen-page, 3,778-word draft, including six citations and some additional references. The overall quality is satisfactory, although there is room for improvement in the number of cited sources. This article successfully integrates complex and contradictory concepts in an organic way, and even discovers some new connections that I had not anticipated. It only cites high-quality academic sources, and the citations include accurate quoted content. Although I cannot fully guarantee the accuracy of all the content (but I did not find any obvious errors), if this were the work of a freshly minted doctoral student, I would be satisfied with their performance.
This AI citation quality milestone marks a significant progress. Citations are no longer the common "hallucinations" or erroneous citations of papers, but legitimate, high-quality academic sources, including the groundbreaking research of my colleagues Saerom (Ronnie) Lee and Daniel Kim. When I click on the citation links, they not only point to the relevant papers, but often directly jump to the specific highlighted excerpts. Although there are still some limitations - the AI can only access content it can find and read within a few minutes, and paid-wall articles remain inaccessible - this represents a fundamental leap in AI's handling of academic literature. For the first time, an AI is not just summarizing research, but actively engaging with it in a manner akin to human academic research.
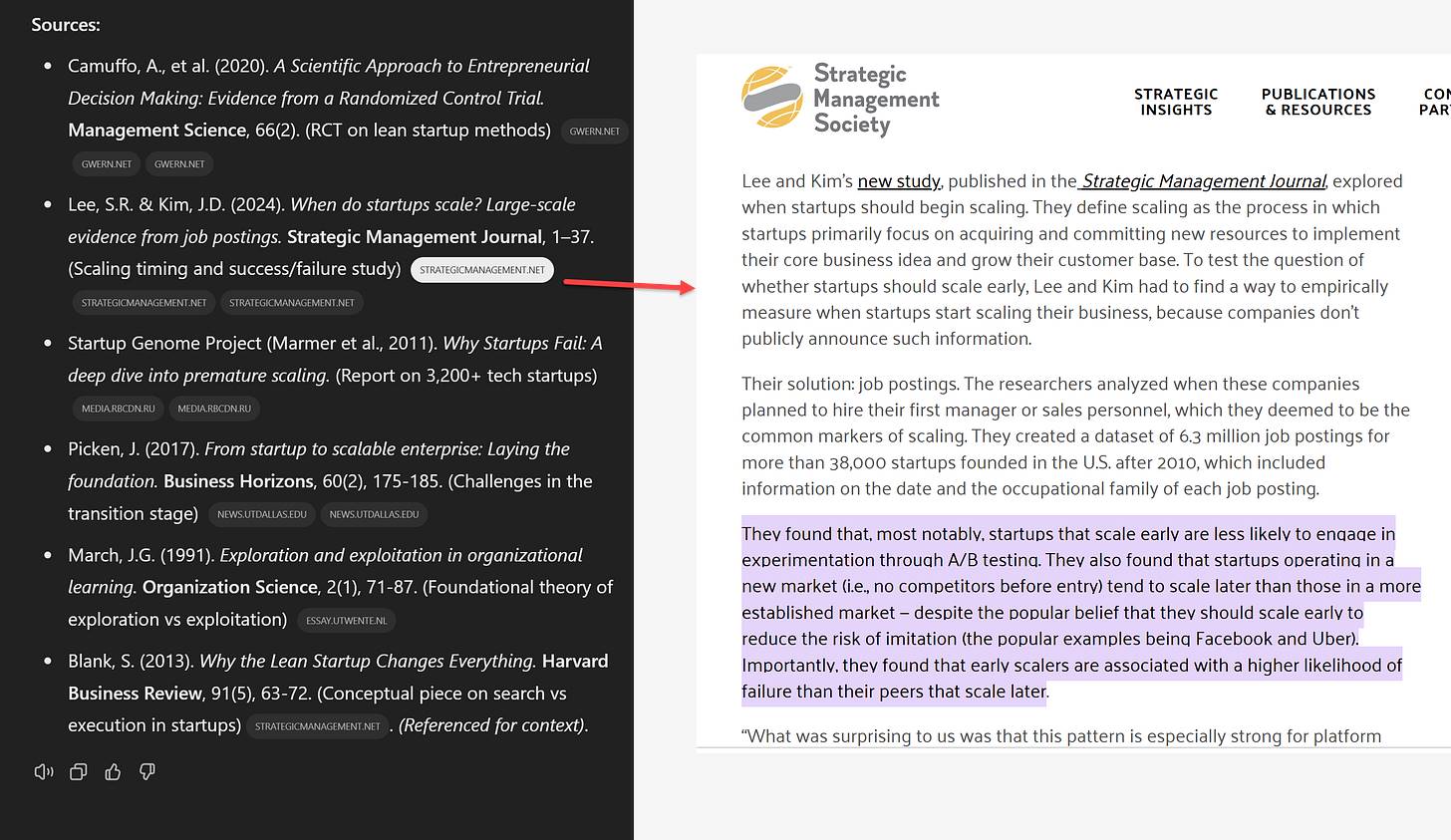
It is worth noting that Google also launched a product of the same name, Deep Research (sigh). Google's system provides more citations, but the quality of the sources is uneven, often a mix of various websites (the inability to access paid information and books is a challenge for all intelligent agents). Unlike the OpenAI research agent, Google's system seems to collect all documents at once, rather than gradually acquiring them through exploratory discovery. Furthermore, since Google's product is currently based on the old Gemini 1.5 model (lacking reasoning capabilities), its summaries are more superficial, although overall they are still solid and without obvious errors. It could be said that its performance is more akin to that of an excellent undergraduate student.
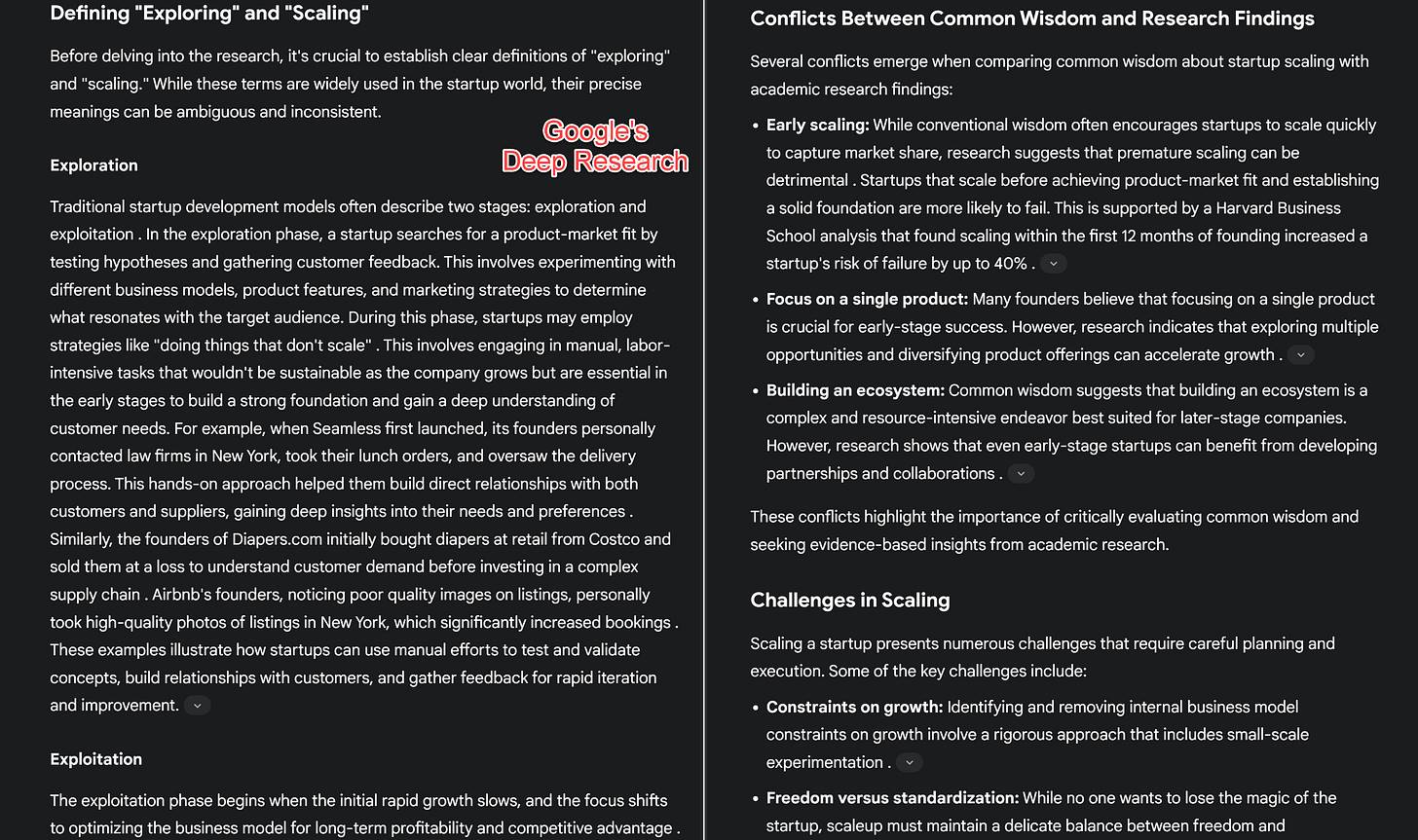
To understand this more clearly: both the OpenAI and Google research agents can complete tasks that typically require hours of human work. The difference is that the OpenAI system has reached a level of analysis approaching that of a doctoral research, while the Google system is more like the work of an excellent undergraduate. In OpenAI's official statement, they made some bold claims and used charts to show that their agent can handle 15% of high-economic-value research projects and 9% of extremely high-value research projects. Although the specific methodologies behind these data are not publicly disclosed, and a certain degree of skepticism is warranted, my actual experience of using it suggests that these claims are not entirely exaggerated. Deep Research can indeed complete complex and valuable analyses in a matter of minutes, rather than hours. Considering the pace of technological progress, I believe Google will not allow this gap to persist for long. In the coming months, we may see a rapid improvement in the capabilities of research agents.
Synergistic Technological Development
Based on current trends, the technologies being built by major AI labs are not simply pieced together, but are achieving higher efficiency through mutual interaction. Reasoning systems (Reasoners) provide powerful logical analysis capabilities, while agent systems endow these reasoning capabilities with the ability to take concrete actions. Currently, we are in the era of narrow-domain agents, such as Deep Research, which focus on specific tasks, as even the most advanced reasoning systems today have not yet reached the requirements for general autonomous capabilities. However, "narrow domain" does not mean limited - these systems are already capable of completing complex tasks that previously required high-paid expert teams or professional consulting firms.
Of course, this does not mean that experts and consulting firms will be replaced. On the contrary, as they shift from directly executing work to coordinating and verifying the output of AI systems, their professional judgment will become even more important. But the goals of AI labs go far beyond this. They aim to crack the problem of general-purpose intelligent agents through more powerful models, transforming them from narrow-domain tasks to true autonomous digital labor. These agents would not only be able to autonomously browse the web, but also handle various forms of data (such as text, images, and audio) and take meaningful actions in the real world. Although the performance of Operator shows that we have not yet fully achieved this goal, the success of Deep Research has already demonstrated that we are steadily progressing in this direction.